Prediction is useless unless prevented
Churn prediction refers to predicting when a user is going to leave the game. No matter how accurate your prediction model is, it is useless if you cannot prevent users from leaving. If you know that a specific user is going to leave on D4 but don’t know how to prevent it, all you can do is watch as it happens. In fact, many churn prediction models stop at prediction and have no actual preventive effect. 7 years ago, a game data analyst introduced his churn prediction model in his blog and added the following.
“It is difficult (for churn prediction) to improve services or add more fun. While it is useful as a user management tool from a marketing perspective, it does not give answers to why users are leaving, what should be fixed, nor how to fix them.”
(Source: https://boxnwhis.kr/2015/02/09/predict_churns.html)
Wouldn’t it be different if it is possible to not only predict churn but also know the causes and content causing users to leave?
Utilizing causes behind churn
How would it be if you knew why a user was leaving your game at a certain point? What would you do if you knew that the reason why a user was leaving on D4 was because he kept failing after choosing to stick to an underdog character?
You could take action so that the user can have a taste of victory before deciding to leave. For instance, you can send an in-game message to the user before D4 to let him know how to strengthen his character or switch to a stronger one. (You can make your suggestion more enticing through quests or rewards.) If the user plays the game as recommended, there is a far lower chance of him leaving the game on D4.
Utilizing churn zones
Let’s say you are aware of the part of your game that is causing a user to leave. You can change it to lower the churn rate.
Learn more about “Full analysis” ▶
By analyzing the churn rate for each stage, you can identify and fix problematic stages. If the churn rate becomes significantly higher at Stage 11-2, it means that this stage is triggering users to leave. If the users were leaving because of the high level of difficulty, the stage can be made easier to improve the retention rate.
What are some tools that support analysis by stage/level?
Read more about “Finding the right game analysis tool”
Using log data to determine churn causes and zones
To identify the causes behind churn, you must examine user behavior through log data. Users’ gameplay patterns and churn zones can be determined by analyzing each user’s level-up, stage clear, skill/character building, virtual currency acquisition/use, and item acquisition/use. Through the integration of behavioral economics, it is possible to learn how users are inclined to behave, what they are looking for, and why they leave the game. Read more about “Game log data collection for beginners”
Learn more about "The Basics of Game Logging: The 5W1H" ▶
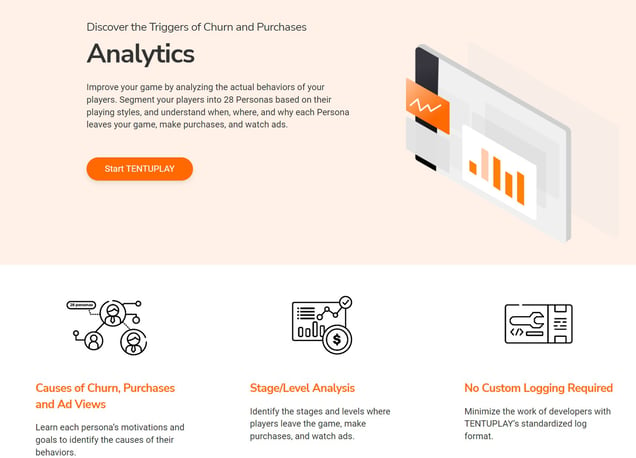
All types of data analysis, including churn analysis, are intended to support effective decision-making, create better games, and improve revenue based on objective evidence. Manage users more effectively and improve your game by analyzing the reasons for churning and zones with high churn rates.
Go to the TentuPlay
Go to the Free Trial